By: Sadequl Hussain | Comments (5) | Related: More > Big Data
Problem
We are already running a large data warehouse for our business, but now the management wants us to build a data lake. What's the difference? Where will we use it? Who will use it?
Solution
A data lake is a centralized location for storing an organization's data assets in their native form. Ideally, these data assets are collected from every touch point of the business and stored with the intention of analyzing in future. The purpose of capturing every data element of interest is to ensure business can use it to gain competitive market advantage. Ever since the start of modern computing, databases have been used for this purpose. Data lakes are a natural extension of databases - and later data warehouses – based on the variety of data and how it's stored or used.
Let's a say a business decides to capture information about its client interactions. This has been the role of CRM (Customer Relationship Management) applications for a long time. Users would record sales prospective, customer feedback and other information in a CRM database. Typically, the database would be relational with predefined tables representing customer and associated entities.
Modern businesses interact with customers in many different ways though: there may be a brick-and-mortar call center using a CRM; but then there will be one or more websites with their feedback forms, direct e-mails, e-commerce store, mobile apps, social media presence or business partner channels. All these are valuable sources of information which can provide a 360 view of the customer. To maintain a competitive advantage in the market, the business will need to capture information from all these outlets.
But not every piece of information can be saved in a database. Some data would be highly unstructured, like images (think of users sending faulty product images); some may be semi-structured, like social media feeds or XML documents. It's impossible to store every type of data in one single database and that's where a data lake can help.
In its basic form, a data lake is nothing but a huge pool of storage where data can be saved in its native, unprocessed form, without any transformation applied. For example, a data lake can store both nightly batches of CSV files (which is structured data) offloaded from the CRM and streaming feeds from the social media channel. The same data lake could be hosting semi-structured customer satisfaction survey files sent by third-party vendors.
How are Data Lakes Different from Data Warehouses?
So now we know a data lake holds all types of information a business deems strategically important, and the information is stored in its native, raw form.
This is not the case the with a data warehouse. First off, data warehouses typically store relational data, which is structured. There are tables in a data warehouse and those tables have relationships and can follow data models like snowflake or star schema.
Secondly, a data warehouse hosts only a subset of data from different sources. For example, a data warehouse can get its data from sales, product, customer and finance database systems, but it may skip any feeds from HR and payroll systems. In other words, data warehouses are purpose-built, meant to answer a specific set of questions. To cater this, source data is cleansed and processed before loading into the data warehouse.
Thirdly, data in a data warehouse may be aggregated in nature. Instead of raw transaction data, aggregated values are used for decision making.
Fourthly, data in a data lake is saved in perpetuity, regardless of use in immediate or near future. The notion here is "store everything; we may need it for future analysis". This is possible because data lakes are typically backed by large amount of storage at low-cost (more on that later).
In contrast, data warehouses are usually run on premium quality, IO-optimized storage for maximum performance. That's why storage for a data warehouse is not cheap, and to preserve performance, a data warehouse does not retain unlimited data: it's usually deleted or archived after an expiration period. Very large data warehouses can use techniques like data marts, partitioning, sharding or federated servers.
Use Cases for Data Lakes vs. Data Warehouses
The use cases for data lakes and data warehouses are quite different as well. There can be more than one way of transforming and analyzing data from a data lake. It may or may not need to be loaded into a separate staging area. For example, CSV files from a data lake may be loaded into a relational database with a traditional ETL tools before cleansing and processing. On other hand, image or video data could be directly analyzed from the lake by a machine learning algorithm. Generally, data from a data lake requires more pre-processing, cleansing or enriching.
This is not the case with data warehouses. Data in a warehouse is already extracted, cleansed, pre-processed, transformed and loaded into predefined schemas and tables, ready to be consumed by business intelligence applications.
That's the reason users and use cases of data lakes and data warehouses are different. Data lakes are often used in big data operations like data mining or machine learning for finding patterns, building predictive models or other complex high value outputs. The users are typically data scientists or advanced data analysts. Tools are typically related to the Hadoop ecosystem like Apache Spark or Hive.
Data warehouse use cases are mainly restricted to business intelligence, reporting, and visualizations with dashboards. The main technical users of a data warehouse are data analysts, report designers or sometimes data scientists, and end users are business decision makers.
This does not necessarily mean data lakes and data warehouses are two isolated business assets. In fact they are supposed to complement each other. For example, raw data in a data lake could be first level assets for a company. A process could load this data in a staging environment, transform it into relational data, aggregate it and then load it in the data warehouse. This transformed data then becomes a second level data asset. Further processing and enriching could be done in the warehouse, resulting in the third and final value-added asset. This final form of data can be then saved back to the data lake for anyone else's consumption.
Schema on Read vs. Schema on Write
Another difference between a data lake and a data warehouse is how data is read. A data lake hosts data in its raw format without any schema attached to it. Schema is only applied when data is read from the lake. This is called schema on read.
For example, let's say a data lake has a collection of many thousand JSON files. These files may not follow any particular schema, they may be many levels deep, but they may also have some common fields. A data scientist can extract only those common fields from each file and populate a table. In this case, the data scientist writes a query to parse the JSON files, extract the field values and populate a table. In other words, a schema is applied on the source files when data is actually read.
Data warehouse schemas are predefined. A schema consists of multiple tables and their fields, data types, constraints and relationships. The schema is designed and developed with business rules in mind and tested for functionality before data is loaded into it. When data is written to a data warehouse, the process can be called "scheme on write" because the write operation is conforming to the already existing schema, otherwise it will be rejected. When data is read from the warehouse, applications do not impose any schemas of their own.
Storage
Let's talk about data lake storage.
Data in a data lake is slow moving. It's not meant for immediate access, but it needs very large storage space. Cloud-based low-cost object storage mediums are therefore perfect fit for data lakes. If we consider Amazon Web Services (AWS), Simple Storage Service (S3) is an ideal solution. Similarly for Microsoft Azure, the storage could be Azure Data Lake Storage. For Google Cloud Platform, it could be Google Cloud Storage.
Data lakes can be also hosted on Hadoop clusters. In this case data is ingested into the Hadoop File System (HDFS) and remains close to the compute power of the data nodes. Total storage capacity of the cluster is the storage available for the data lake.
ELT vs. ETL
Transforming data is not so much a priority in data lakes as much is loading data. Typically, data pipelines for a data lake extract data from source systems and loads that into target as quickly as possible. ELT (Extract, Load and Transform) tools are therefore ideally suited for this. Many ELT tools can connect to data lake storage systems natively. The transformation part generally varies between data consumers, so there can be many different types of transformation use cases. Generally, transformation is done by end-user applications connecting to the data lake. Using only the "E" and "L" of ELT also means data lake pipelines are simple and inexpensive.
Data Warehouses typically depend on ETL (Extract, Transform and Load) where one or more ETL packages run within one or few dedicated compute resources. This compute layer extracts data, transforms it, and then loads it into the data warehouse. The transformation processes for data warehouses are well defined, represent strict business rules, and repetitive in nature.
Another difference between data lake ELT and data warehouse ETL is how they are scheduled. With data lakes, there may (or may not) be, scheduled loading and transformation processes. Some transformations can be one-off or ad-hoc. Data warehouse transformations are almost always scheduled.
Data Lake Challenges and Best Practices
Like any other technology solution, data lakes come with its own set of challenges and best practices. Here are a few:
Data Governance
Businesses often start data lake projects by assuming it needs to store every piece of data it comes across. This is natural thinking, but it also means the lake could be containing unnecessary information which will never be used. Unless checked, a data lake risks becoming a swamp - a dumping ground for everyone's data.
Storage might be cheap, but storing massive amounts of unnecessary data also means:
- The time to seek out relevant information will also increase
- There will be no definite catalog of data in the lake
- Other issues like data duplication or security will need to be addressed (discussed next)
Data stewards or data architects therefore need to address this proactively from the very onset of the project. The company needs to decide what type of information it's going to store in the lake, where it will be sourced from, how it will be stored and who will be the custodians and consumers of that data.
For example, if a business offers managed IoT service for its customers, saving online ad clickstream data in the lake may not be as important as saving all the IoT data.
Data Duplication
This is another pitfall of poor data governance. When people know about a data lake, they quickly start to build their own "areas" within the lake: silos of data related to their own teams or departments, unaware that another team might be saving the exact information in different form somewhere else in the lake. When a consumer searches for a data item, it may find the same piece of data in two different places, not knowing which version is the latest and more trustworthy.
There are two ways to address this challenge:
- Data stewards and data architects can build specific areas in the lake for different data sources. These areas should be accessible by every team involved in the data's lifecycle. The existence of the area is then communicated to these teams.
- Maintaining appropriate metadata for different sets of data. For example, a data lake folder containing aggregated information from accounts payable system can have a metadata file showing the source server name, the date of loading, the user account that loaded the data and the accounting period.
Data Security
Security is a complex thing to manage in data lakes. Data warehouses usually have DBAs who build security models around databases, schemas or tables for specific sets users, groups or applications and their access requirements. Data lakes are quite different. They have varying - and often ad-hoc - needs of access from users, applications or even external parties. And because it's implemented on top of an object storage system, there is no DBA to manage security, no command to run for restricting access.
There are few ways to address this issue:
- The same team responsible for the organization's data security should be entrusted with protecting the data lake. This may be the DBA team or the operations team.
- The security team will provide read access to required files or folders to relevant users as and when necessary.
- There should be only specific accounts – preferably application accounts – that can load data in the lake.
- The data lake will not store sensitive data like personal or financial information in its original form. It will be masked before storing. The application responsible for using the data will need to decipher it.
- If using cloud-based storage, all data should be encrypted with one or more keys that are regularly rotated.
- There should be adequate measures for backing up critical pieces of data or at least versioning them. For example, Amazon Web Service S3 storage allows long time retention of data at lower cost in Amazon Glacier and enable versioning of files in a bucket.
Data Toolsets
With a wide variety of data types and their use-cases, data lake users can quickly start using tools of their own choice. This can quickly grow into an organization-wide proliferation of non-standard (and often vulnerable) tools and practices. It's therefore necessary to standardize on a set of tools for accessing the data lake and writing to it.
For example, it may be stipulated that data scientists only use a particular type of notebook technology, use specific languages like R or Python and use a set of data manipulation libraries. Using the same model, data analysts could be asked to use one or two specific SQL query tools that are licensed and ETL developers could be trained to use a standard integration tool.
Data Lake Adoption
Perhaps more challenging than implementing security is to actually make people use the data lake. In today's world, information should be democratized, people should be able to access relevant data without any hassle or too many hoops. If users see the data lake as an opaque, complex piece of technology, they will quickly turn away from it, defeating the purpose of a unified platform.
It's therefore necessary to build some self-service capability for different groups of users. This can start with a simple awareness program where users are trained on the data lake's existence, it's purpose, the business value it offers and how to use it with existing tooling. Further down the journey, the company can invest on self-service portals for ad-hoc searching and query building.
Conclusion: Some Data Lake Technologies and Vendors
Hopefully this article has given some background on data lakes and their best practices. We recommend users familiarize themselves with tools and technologies for data lake solutions offered by different vendors. Here are some starting points:
- AWS Lake Formation is a service from Amazon Web Service for creating a secured data lake with minimal manual configuration. Currently it's in preview mode.
- Microsoft Azure Data Lake consists of a number of underlying tools for building a data lake.
- IBM and Hortonworks offer joint solutions for data lake on the Hadoop ecosystem.
Next Steps
- Find if your company is currently using a data lake. If not, can it benefit from a data lake? Investigate if there are important data elements currently missing from your data stores which can add value to the business.
- Learn more about data lake technologies offered by different vendors. This can include storage vendors, data integration vendors, database vendors, BI tool vendors.
- If your company already has a data lake, identify its users, use cases, tools and any current challenges.
- Understand your organization's data governance practices and how the data is shared.
About the author
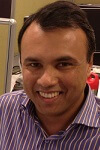
This author pledges the content of this article is based on professional experience and not AI generated.
View all my tips