By: Arshad Ali | Comments (38) | Related: 1 | 2 | 3 | 4 | > Integration Services Best Practices
Problem
SQL Server Integration Services (SSIS) has grown a lot from its predecessor DTS (Data Transformation Services) to become an enterprise wide ETL (Extraction, Transformation and Loading) product in terms of its usability, performance, parallelism etc. Apart from being an ETL product, it also provides different built-in tasks to manage a SQL Server instance. Although the internal architecture of SSIS has been designed to provide a high degree of performance and parallelism there are still some best practices to further optimize performance. In this tip series, I will be talking about best practices to consider while working with SSIS which I have learned while working with SSIS for the past couple of years.
Solution
As mentioned above, SSIS is the successor of DTS (of SQL Server 7/2000). If you are coming from a DTS background, SSIS packages may look similar to DTS packages, but it's not the case in reality. What I mean is, SSIS is not an enhancement to DTS but rather a new product which has been written from scratch to provide high performance and parallelism and as a result of this it overcomes several limitations of DTS.
SSIS 2008 has further enhanced the internal dataflow pipeline engine to provide even better performance, you might have heard the news that SSIS 2008 has set an ETL World record of uploading 1TB of data in less than half an hour.
The best part of SSIS is that it is a component of SQL server. It comes free with the SQL Server installation and you don't need a separate license for it. Because of this, along with hardcore BI developers, database developers and database administrators are also using it to transfer and transform data.
Best Practice #1 - Pulling High Volumes of Data
Recently we had to pull data from a source table which had 300 millions records to a new target table. Initially when the SSIS package started, everything looked fine, data was being transferred as expected but gradually the performance degraded and the data transfer rate went down dramatically. During analysis we found that the target table had a primary clustered key and two non-clustered keys. Because of the high volume of data inserts into the target table these indexes got fragmented heavily up to 85%-90%. We used the online index rebuilding feature to rebuild/defrag the indexes, but again the fragmentation level was back to 90% after every 15-20 minutes during the load. This whole process of data transfer and parallel online index rebuilds took almost 12-13 hours which was much more than our expected time for data transfer.
Then we came with an approach to make the target table a heap by dropping all the indexes on the target table in the beginning, transfer the data to the heap and on data transfer completion, recreate indexes on the target table. With this approach, the whole process (by dropping indexes, transferring data and recreating indexes) took just 3-4 hours which was what we were expecting.
This whole process has been graphically shown in the below flow chart. So the recommendation is to consider dropping your target table indexes if possible before inserting data to it specially if the volume of inserts is very high.
%20-%20Best%20Practices%20-%20Part%201%20-%20img1.jpg)
Best Practice #2 - Avoid SELECT *
The Data Flow Task (DFT) of SSIS uses a buffer (a chunk of memory) oriented architecture for data transfer and transformation. When data travels from the source to the destination, the data first comes into the buffer, required transformations are done in the buffer itself and then written to the destination.
The size of the buffer is dependant on several factors, one of them is the estimated row size. The estimated row size is determined by summing the maximum size of all the columns in the row. So the more columns in a row means less number of rows in a buffer and with more buffer requirements the result is performance degradation. Hence it is recommended to select only those columns which are required at destination.
Even if you need all the columns from the source, you should use the column name specifically in the SELECT statement otherwise it takes another round for the source to gather meta-data about the columns when you are using SELECT *.
If you pull columns which are not required at destination (or for which no mapping exists) SSIS will emit warnings like this.
[SSIS.Pipeline] Warning: The output column "SalariedFlag" (64) on output "OLE DB Source Output" (11) and component "OLE DB Source" (1) is not subsequently used in the Data Flow task. Removing this unused output column can increase Data Flow task performance.
[SSIS.Pipeline] Warning: The output column "CurrentFlag" (73) on output "OLE DB Source Output" (11) and component "OLE DB Source" (1) is not subsequently used in the Data Flow task. Removing this unused output column can increase Data Flow task performance.
Beware when you are using "Table or view" or "Table name or view name from variable" data access mode in OLEDB source. It behaves like SELECT * and pulls all the columns, use this access mode only if you need all the columns of the table or view from the source to the destination.
Tip : Try to fit as many rows into the buffer which will eventually reduce the number of buffers passing through the dataflow pipeline engine and improve performance.
Best Practice #3 - Effect of OLEDB Destination Settings
There are couple of settings with OLEDB destination which can impact the performance of data transfer as listed below.
Data Access Mode - This setting provides the 'fast load' option which internally uses a BULK INSERT statement for uploading data into the destination table instead of a simple INSERT statement (for each single row) as in the case for other options. So unless you have a reason for changing it, don't change this default value of fast load. If you select the 'fast load' option, there are also a couple of other settings which you can use as discussed below.
Keep Identity - By default this setting is unchecked which means the destination table (if it has an identity column) will create identity values on its own. If you check this setting, the dataflow engine will ensure that the source identity values are preserved and same value is inserted into the destination table.
Keep Nulls - Again by default this setting is unchecked which means default value will be inserted (if the default constraint is defined on the target column) during insert into the destination table if NULL value is coming from the source for that particular column. If you check this option then default constraint on the destination table's column will be ignored and preserved NULL of the source column will be inserted into the destination.
Table Lock - By default this setting is checked and the recommendation is to let it be checked unless the same table is being used by some other process at same time. It specifies a table lock will be acquired on the destination table instead of acquiring multiple row level locks, which could turn into lock escalation problems.
Check Constraints - Again by default this setting is checked and recommendation is to un-check it if you are sure that the incoming data is not going to violate constraints of the destination table. This setting specifies that the dataflow pipeline engine will validate the incoming data against the constraints of target table. If you un-check this option it will improve the performance of the data load.
Best Practice #4 - Effect of Rows Per Batch and Maximum Insert Commit Size Settings
Rows per batch - The default value for this setting is -1 which specifies all incoming rows will be treated as a single batch. You can change this default behavior and break all incoming rows into multiple batches. The allowed value is only positive integer which specifies the maximum number of rows in a batch.
Maximum insert commit size - The default value for this setting is '2147483647' (largest value for 4 byte integer type) which specifies all incoming rows will be committed once on successful completion. You can specify a positive value for this setting to indicate that commit will be done for those number of records. You might be wondering, changing the default value for this setting will put overhead on the dataflow engine to commit several times. Yes that is true, but at the same time it will release the pressure on the transaction log and tempdb to grow tremendously specifically during high volume data transfers.
The above two settings are very important to understand to improve the performance of tempdb and the transaction log. For example if you leave 'Max insert commit size' to its default, the transaction log and tempdb will keep on growing during the extraction process and if you are transferring a high volume of data the tempdb will soon run out of memory as a result of this your extraction will fail. So it is recommended to set these values to an optimum value based on your environment.
Note: The above recommendations have been done on the basis of experience gained working with DTS and SSIS for the last couple of years. But as noted before there are other factors which impact the performance, one of the them is infrastructure and network. So you should do thorough testing before putting these changes into your production environment.
Next Steps
- Review OLEDB Destination on MSDN.
- Read other SSIS Best Practices Tips
About the author
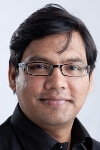
This author pledges the content of this article is based on professional experience and not AI generated.
View all my tips